As artificial intelligence (AI) continues to revolutionize industries, companies are eager to harness its potential for innovation and competitive advantage. However, many organizations overlook a critical prerequisite for successful AI implementation: a robust and reliable data foundation. This article explores the importance of preparing your data to be AI-ready, highlighting key challenges and strategies for building a solid data infrastructure. It is intended for software engineers, technical leaders, and decision-makers seeking to unlock the full potential of AI in their organizations.
The Growing Investment in AI and Data Management
According to the latest Deloitte State of Gen AI Report, 75% of organizations have increased their technology investments in data life cycle management due to generative AI. While many companies believe they are mature in data management, unforeseen issues often arise when scaling AI initiatives from proof of concept to full deployment. In fact, 55% of organizations have avoided certain AI use cases because of data-related issues.
Key Concerns Impacting AI Readiness
Despite the enthusiasm for AI, organizations face several concerns:
- Data Sensitivity: Are we risking exposure of sensitive customer or client information when using AI models? A significant 58% of organizations express high levels of concern about this.
- Data Privacy and Security: How secure is our data when leveraged by AI technologies? Data privacy issues worry 58% of organizations, while 57% are apprehensive about data security risks.
- Data Quality: Do we have the right data, and is it accurate? Inconsistent or poor-quality data can lead to inaccurate AI predictions and insights.
These concerns highlight the necessity of addressing data management challenges to fully leverage AI technologies.
What makes data truly AI-ready? For AI systems to deliver meaningful and reliable outcomes, they require data that meets specific criteria. Imagine trying to build a house on an unstable foundation—the same principle applies to AI and data. Without a solid data foundation, your AI initiatives are likely to falter.
Characteristics of AI-Ready Data:
- Comprehensive and Well-Documented: Does your data include comprehensive metadata, such as schema definitions and semantic information? Detailed documentation enables both humans and AI models to interpret and reason about the data effectively, ensuring clarity and consistency across analyses.
- Clean and Well-Structured: Is your data free from errors and inconsistencies, and is it systematically organized? Clean, structured data facilitates easy querying, reduces the time spent on data preparation, and enhances the overall efficiency of AI processes.
- Accurate and Reliable: Can you depend on your data to make informed decisions? Ensuring data accuracy is crucial for generating precise AI predictions and maintaining the integrity of your analytical outcomes.
- Longevity: Has your data schema been consistently used over an extended period to satisfy various use cases? Longevity ensures that your data structures are reliable and resistant to breaking changes, providing a stable foundation for long-term AI initiatives without compromising flexibility.
- Extensibility: Can your data framework accommodate specialized business needs that may arise unexpectedly? Extensibility allows your data systems to integrate custom fields and adapt to unique requirements, ensuring that your AI solutions remain relevant and effective as your business evolves.
By focusing on these characteristics, organizations can ensure their data is ready to power successful AI applications.
Challenges in Achieving AI-Ready Data
Despite recognizing the importance of data readiness, many organizations face significant hurdles. Have you ever wondered why your AI projects aren’t delivering the expected results? The answer often lies in the underlying data.
- Data Fragmentation: Is your data scattered across multiple integrations, including data lakes, warehouses, and databases? Such fragmentation can impede AI models from accessing the full spectrum of information needed for accurate insights. Disparate integrations make it challenging to maintain a cohesive data ecosystem, limiting the effectiveness of AI-driven initiatives.
- Lack of Contextualization: Without proper categorization and metadata, could your AI systems misinterpret data? Without context, AI may draw erroneous conclusions.
- Consistent Formatting: Is your data formatted uniformly across all sources? Consistent data formatting ensures that AI models can process and analyze information without encountering discrepancies that could lead to errors.
- Standardized Data Models: Do you have a standardized data model in place? A unified data model facilitates seamless data integration and interpretation, allowing both humans and AI systems to understand and utilize the data effectively.
- Proactive Storage and Processing Planning: Have you considered storage and processing scenarios for your data ahead of time? Anticipating future data needs and processing requirements ensures that your infrastructure can support scalable AI initiatives without unforeseen bottlenecks.
- Outdated or Irrelevant Data: Are you relying on redundant, obsolete, or trivial (ROT) content? Using outdated information can compromise the validity of AI-generated insights.
According to a survey, 70% of organizations adopt hybrid cloud storage practices, with nearly half managing multiple cloud solutions. Additionally, only 23% have systems that provide real-time access to enterprise resource planning (ERP) data for decision-making. These statistics highlight the widespread nature of these challenges.
Strategies for Building a Robust Data Foundation
So, how can organizations overcome these obstacles and prepare their data for AI success? Building a solid data foundation requires a strategic approach that addresses the root causes of data challenges.
Centralizing and consolidating your data is a crucial first step. By breaking down data silos and unifying data across departments and systems, you create a single source of truth. Have you considered how centralizing your data can enhance accessibility and efficiency? When AI models have seamless access to all relevant information, they can generate more accurate and comprehensive insights.
Enhancing data quality and context is equally important. Improving data quality practices involves investing in processes and tools that ensure your data is clean, accurate, and reliable. Adding context through metadata—such as timestamps, location information, and document classifications—enables AI systems to interpret data correctly. Could integrating complementary data, like customer demographics, unlock deeper insights for your organization?
As regulatory landscapes evolve, updating data governance and security measures becomes vital. However, it’s not just about current compliance—anticipating future scenarios is equally important.
Imagine a scenario where new compliance requirements mandate the redaction or expunging of specific data. How prepared is your data infrastructure to handle such changes without disrupting your AI initiatives?
At Data Layer, we designed our platform with these future scenarios in mind. Our centralized data pane ensures that compliance and regulatory mechanisms surrounding your business data are easily manageable. Whether you need to redact sensitive information or adapt to new regulations, Data Layer provides the flexibility to implement these changes seamlessly.
Ensuring data relevance and timeliness is another key strategy, but it’s important to balance this with the longevity of your data.
Implementing lifecycle policies helps manage the life cycle of your data without necessarily purging it. Historical data can provide valuable insights and enhance the accuracy of AI models by offering a comprehensive view over extended periods.
- Retention for Insights: Maintaining data over longer periods allows for more robust model training, capturing trends and patterns that short-term data might miss.
- Cost-Effective Storage: Streamlined data ecosystems that retain relevant historical data while managing storage costs effectively.
- Enhanced Decision-Making: Leveraging both current and historical data ensures that your AI models are informed by a rich dataset, improving the reliability of their predictions.
Introducing Data Layer: Preparing Your Data for AI Success
Navigating the complexities of data preparation can be daunting. This is where Data Layer comes into play. Data Layer offers a comprehensive solution to help organizations establish a robust data foundation for AI initiatives. By ensuring your data is consistent, reliable, and AI-ready, Data Layer enables you to drive transformative insights and innovation.
How Data Layer Can Help
- Unified Data Integration: Data Layer unifies disparate data sources into a cohesive, centralized system, eliminating fragmentation.
- Enhanced Data Quality Management: It implements rigorous validation and cleansing processes to maintain high data standards.
- Metadata Enrichment: Data Layer adds comprehensive metadata and documentation, providing context and facilitating easier data interpretation.
- Flexible Data Management: The solution adapts to various environments—on-premises or cloud-based—offering scalable solutions tailored to your needs.
- Centralized Policy Management: It ensures consistent compliance with data governance policies across the organization.
By partnering with Data Layer, you can overcome data-related obstacles and fully realize the benefits of AI technologies.
Remember, a robust data foundation doesn’t just support your current AI initiatives; it future-proofs your organization for the evolving data landscape. With partners like Data Layer, you can navigate this journey with confidence, ensuring your data is truly AI-ready.
Discover How to Make Your Data AI-Ready – Schedule a Free 45-Minute Session!
Take the next step towards transforming your data strategy. Schedule a free 45-minute session with our data experts to discover how Data Layer can optimize your data for AI-driven success. In this personalized consultation, we’ll assess your current data infrastructure, identify key areas for improvement, and provide actionable insights tailored to your unique business needs.
References:
- Deloitte. (2024). State of Gen AI Report.
- G2. (2023). Hybrid Cloud Storage Practices Survey.
- IBM. (2024). Cost of a Data Breach Report.
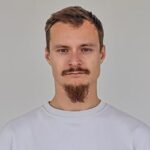